Welcome to the page of the Kietzmann Lab, which is part of the Institute of Cognitive Science, University of Osnabrück. We investigate principles of neural information processing using tools from machine learning and deep learning, applied to neuroimaging data recorded at high temporal (EEG/MEG) and spatial (fMRI) resolution. Please contact us with any questions or paper requests, and follow Dr. Kietzmann on twitter (@TimKietzmann) for latest lab updates.
Please reach out to us if you are interested in joining the lab and see our page on equity, diversity, and inclusion for further information.
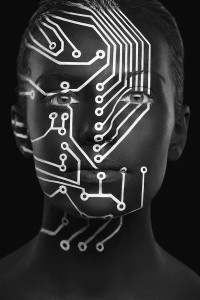
Research Interests
Cognitive Neuroscience meets Machine Learning. Our research group aims to understand the computational processes by which the brain and artificial agents can efficiently and robustly derive meaning from the world around us. We ask how the brain acquires versatile representations from the statistical regularities in the input, how sensory information is dynamically transformed in the cortical network, and which information is extracted by the brain to support higher-level cognition. To find answers to these questions, we develop and employ machine learning techniques to discover and model structure in high-dimensional neural data.
As a target modality, we focus on vision, the most dominant of our senses both neurally and perceptually. To gain insight into the intricate system that enables us to see, the group advances along two interconnected lines of research: machine learning for discovery in neuroscience, and deep neural network modelling. This interdisciplinary work combines machine learning, computational neuroscience, computer vision, and semantics. Our work is therefore at the heart of the emerging fields of neuro-inspired machine learning and cognitive computational neuroscience.